Computational Drug Discovery: Increased Applications
We previously discussed how there are software methods to screen for potential drug leads and researchers have been able to increase their drug lead potentials through computational methods. However, finding potential drug leads are not enough in the drug discovery pipeline, and computational drug discovery can be utilized to actually predict and optimize a drug’s metabolism and its pharmacokinetic properties. These properties are important for the optimization of drug delivery without the loss of the drug’s inherent biologic activity.
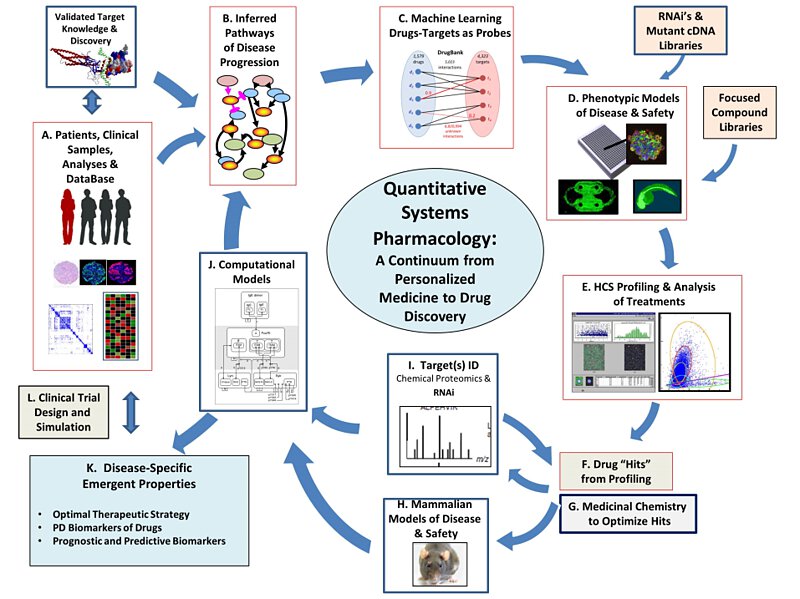
Some of the software used for the optimization of drug leads are other features of the screening software, including AutoDock. Other popular software used to optimize the physical aspects of the drug, including its metabolic properties, includes MetaSite, GOLD, FlexX, DOCK, and C-Score. Researchers will also search reference datasets using methods such as Tripos Topomer Search, Discovery Studio molecular simulation package, or a Gaussian process method for compounds that are similar to the potential lead, track and observe the physical properties of these similar compounds and then determine the probability that a particular compound will fall into a specific functionality class and be effective as a potential drug.2 A quick search on Scientist for pharmacophore modeling lists 38 vendors that provide such services, which can be a great asset to a biotech start up looking to further develop their drug lead. In fact, Scientist lists 46 vendors that provide computational chemistry services, which can potentially assist computationally in various steps of the drug discovery pipeline!
Besides using physical data to screen for and optimize potential drug leads, researchers can use computational methods to actually model clinical trial outcomes and predict potential side effects of a particular lead. This ability is very useful in designing better and more efficient clinical trials for a drug! Depending on the complexity of the clinical trial simulation, software tools such as Excel, MATLAB, or R can be used. However, MONMEM, Pharsight Trial Simulator, and SimCyp are specialized software that support pharmokinetic simulations and can provide flexibility for pharmokinetic modeling. These trial simulations can either inform researchers on the optimal dosage of a particular drug or inform potential new trials of the pitfalls and challenges of designing new clinical trials. Major pharmaceutical companies such as Novartis, Pfizer, and Eli Lily have used clinical trial simulation in their drug development pipelines, and the increased use of computational modeling clinical trials have steadily increased in the past decade.3
Computational drug discovery has truly come a long way from merely improving high throughput screening to potentially predicting the outcome of clinical trials! Computational methods have really revolutionized the drug discovery pipeline. Not only are technology and software improving with the increasing number of researchers interested in computational methods, but perhaps the exciting possibilities in advancing drug discovery is the biggest motivator for computational drug discovery. For those who want to learn where these computational methods have actually advanced drug discovery, stay tuned for next week’s article on the clinical translation of computational drug discovery!
References
- http://www.upddi.pitt.edu/index.php?page=computational-integration
- Sliwoski G, Kothiwale S, Meiler J, Lowe Jr. EW. Computational Methods in Drug Discovery. Pharmacological Reviews (2014) 66 1, 334-395.
- Holford N, Ma SC, Ploeger BA. Clinical Trial Simulation: A Review. Clinical Pharmacology & Therapeutics (2010) 88 2, 166-182.