Exploring the Role of Data Sciences in the Life Sciences, Omics Analytics and Health IT
The life science industry is fortunate to enter the area of big data at a time when other scientific disciplines/industries have paved the way. Big consumers of data (e.g. astronomers, weather forecasters, online retailers and distributors of social media content) have contributed in their own way to establishing large and steady information technology infrastructures on par with the volume of data produced.
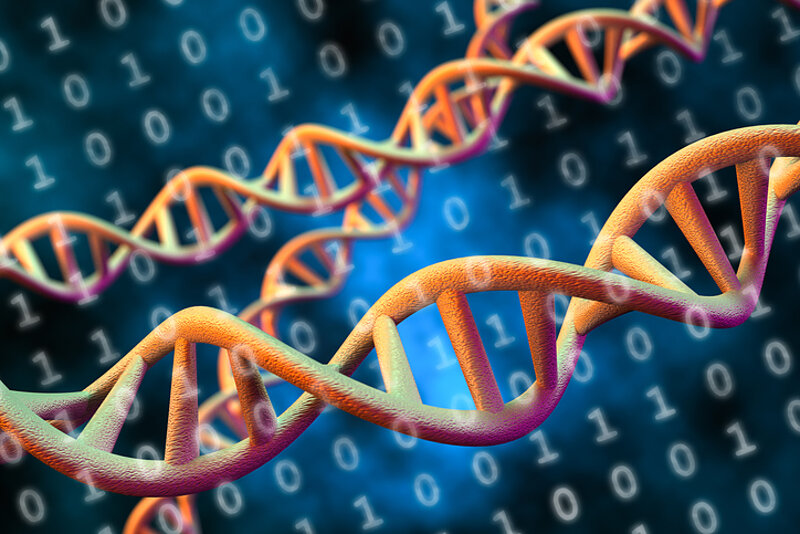
Big data analytics entails newly developed technology mainly concerned with optimizing distributed computing and data storage across thousands of nodes and data centers such as Hadoop and MapReduce, which were co-developed by Yahoo and Google, respectively. The Amazon cloud computing services allow analysts to allocate computation resources on demand with an advanced high-computing operating system (the EC2, Elastic Cloud Computing).
Hence, even though life science organizations are dealing with larger data sets, they are now able to accommodate them with non-capital spending by subscribing to a range of services, from IaaS (Infrastructure as a Service, e.g. AWS, MS Azure, GCG) to SaaS (Software as a Service) to PaaS (Platform as a Service, e.g. Qubloe and IBM Bluemix).
In addition to having access to these IT resources, scientists can leverage machine learning and AI methods developed by someone like Facebook to predict what would be your next click. Instead, these methods can now be turned to figure out what makes a subject most at-risk of contracting a given disease given her/his genotype and lifestyle.
So why not take advantage of optimizing the use of data sciences from the beginning of your research?
I’d like to highlight three subsets of Data Sciences — life sciences data services (discussed above), omics analytics and health IT — and close with an overview of three different organizations to assist scientists in furthering their research.
Omics analytics is primarily involved in capturing, transforming and packaging molecular measurements values. Based on the current status of omics technologies, and also based on its rank as the molecular media of life, DNA is by far the most heavily measured molecules. However, proteomics and metabonomics are not far behind. The latter two also proved to be useful in order to infer molecular networks contributing to a given trait or clinical end-point. For the most part, the variables considered in that area are numeric with the exception of genotype/polymorphism values. Omics analytics include what has historically been considered the realm of bioinformatics, including DNA sequence and gene expression analyses but now encompasses the downstream analyses consisting of the biological interpretation, notably in light of the prior knowledge.
Within the health IT realm, medical research deals with a complete different set of data, including clinical and health care data. Firstly, there is the whole legacy of years of medical research recorded in the literature, e.g. in our venerable U.S. National Library of Medicine just to name one. Secondly, thanks to recent efforts aimed at creating the most cost-effective health care, regulators are promoting the advent of a systemic capture of clinical data in electronic form. While this effort is under way, it creates a wealth of data that would ultimately serve today’s patients but mostly future patients. More data will allow for predictive models of disease inception and/or progression as well as ranking therapeutic procedures that outperform others. Clinicians and researchers alike still face formidable challenges when attempting to take full advantage of this data. The main issue surrounds transforming unstructured free text into data structures relevant to computational environments. A second hurdle is to convince the data source into naming conventions so that quantities of interest that need to be modelled fall into a defined set of values. These activities fall into the generic operations of data curation, which are performed programmatically and via the manual intervention of experts.
To further assist in the advancement and usage of Data Sciences, I’d like to introduce three companies positioned perfectly to help in the three subsets I just described:
- Advaita Bioinformatics is a leading omics analytics organization with in-depth expertise in inferring molecular/biological networks from omics variables as input variables. Advaita has designed methods to infer regulatory circutries from small RNA-seq data sets including miRNA data.
- Arrayo excels in the health IT area with some overlap in the burgeoning life science data services area. For example, Arrayo analysts will extract relevant data from an unstructured free text data source, map them to ontologies (e.g. snomed-ct) and package the results into machine-readable data structures, e.g. the RDF format. The Arrayo team develops on-demand software packages allowing data inter-operability mainly via web-services technologies.
- Incite Advisors provides the means to mine the vast source of data made publicly available through ClinicalTrials.gov. This is a typical case where this resource, i.e. ClinicalTrials.gov data, is useful, only if numerous non-trivial data transformations and formatting are run in order to make it readily consumable by other data mining applications and/or manually interrogated by a clinician. Incite Advisors, through its TrialIO application, structures and processes the data, enabling accurate data mining.